I'm a paragraph. Click here to add your own text and edit me. It's easy.
I'm a paragraph. Click here to add your own text and edit me. It's easy.
YEP ALL THIS STUFF I POSTED ON MY TIMELINE YEARS AGO BEFORE I EVEN KNEW ABOUT THIS BOOK
http://paei.wikidot.com/snowden-d-j-cynefin-sensemaking-framework
The Cynefin (kun-ev’in) project grew out of work at the IBM Institute for Knowledge Management that later migrated to Cardiff University and the Cynefin Centre. Cynefin is a Welsh word meaning ‘our place of belonging’, a place of great meaningfulness for a people. Snowden describes Cynefin as a sensemaking methodology, which differs from earlier knowledge management initiatives in its emphasis on setting up an environment for people to come together and make joint sense of their situation (Kurtz & Snowden, 2003[1]).
Snowden recounts how early knowledge management tended to focus on objectifying tacit knowledge, extracting it from experts and turning it into codified corporate data. Expert knowledge proved not to be entirely extractible in this way. Later approaches still focused on making tacit knowledge explicit, but emphasized the limited usefulness of codification and the important of social processes of knowledge storage, generation and flow. The Cynefin project dispenses with the assumption that knowledge is a thing with a definite rule-like structure, and brings people together to make meaning, initially by building a shared context woven out of shared stories, anecdotes, organizational legends, alternative histories and accounts of phases and events in the organization’s life, and so on. This kind of shared context makes knowledge work vastly more productive and efficient, as evidenced by the speed with which one can explain a process to someone one sees every day, versus to a stranger.
Cynefin practitioners then take teams through a well-defined group process. Teams emerge with more than plans and prescriptions; they also create shared memories and experience. This is an extensive methodology which I do not intend to summarize in its entirety. The main point of contact that I wish to emphasize is the Cynefin framework, which describes four differently ordered/unordered domains describing problem dynamics, plus a fifth area of disorder. These are listed below in PAEI order:
P – Knowable (Ordered – Sense, Analyze, Respond): This order requires pragmatic solutions, analytical thought and scenario planning. There are things we don’t know but could probably figure out. However, we often don’t have time or money to spare for re-inventing this wheel, so we call an expert. Besides expert opinion, trial and error and fact-finding can get us to our goal: to figure out cause-effect relationships and get things done. Sensing data, analyzing it and getting an expert to interpret it and recommend a course of action are good techniques here. Habit can lead us astray, and plans have to remain flexible for updating. Ultimately they will reflect what finally seems to have worked.
A – Known (Ordered – Sense, Categorize, Respond): Problems in this domain are legitimate targets for explicit codification. Cause and effect relationships can be empirically determined and are generally linear. Prediction is possible and issues are objective enough that best practice recommendations are widely accepted. In this category, process reengineering and the explicit codification of structured processes are beneficial and essential. The decision model for this domain includes detecting incoming data, categorizing it and responding according to predetermined practice.
E – Chaos (Unordered – Act, Sense, Respond): In chaos, cause and effect relationships are not discernable. Patterns of turbulence provide the only visible structure to events. Interventions from known domains are not useful and may have caused the present chaos in the first place. Managing this requires a bold and confident leap into the chaos, relying on guts and intuition. Quick action to reduce turbulence and find platforms of relative stability are important, and it may be necessary to establish dominance to accomplish this. This has to be done with ears and eyes wide open, because the results will guide the next hop into the unknown. Done well, desirable patterns of stability will form. This process can also be entered into willingly as an innovation practice.
I – Complex (Unordered – Probe, Sense, Respond): Patterns in this domain emerge from the complex interactions of many different people. Cause and effect relationships are visible, but they are so many that their logic can only be perceived in retrospect, not predicted from the present. A history of this event will be writeable, but the next step is not predictable. There may be a stable pattern for now, but the number of factors at play keep the situation always unpredictably close to major changes. Decisions should be made by sending out probes to assess the prevailing patterns, and seeking multiple perspectives on the significance of unfolding events. Action is best taken by stabilizing and supporting desirable patterns of activity and destabilizing undesirable ones.
The Domain of Disorder: This is the zone for situating conflict among decision-makers. It is the battlefield where proponents favoring solutions from each of the other four zones try to “lay down the law” and control the definition of the problem to match their own interpretation of it. The more contentious the issue, the stronger the desire to pull it towards one’s preferred style of response. Visibly representing this domain in the Cynefin framework and focusing the team on reducing its size on the grid is an important prelude to consensus-building in this methodology.
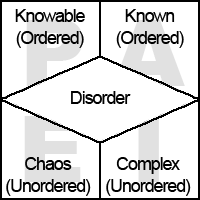
http://paei.wikidot.com/hill-surdu-pooch-anticipatory-planning-support-system
There is a military saying that a plan never survives the first shot. Military planners know and expect that unanticipated events will overtake their plans, forcing commanders into a reactive planning mode during operations. Hill, Surdu and Pooch propose a system architecture called the Anticipatory Planning Support System (APSS) to support planners as they update their plans in real time by helping them anticipate enemy and friendly courses of action as news comes in (Hill et al., 2000; Hill & Surdu, 2001).
APSS is an agent-based decision support system that works with another such system called OpSim (Operationally-focused Simulation, Surdu & Pooch, 2000). It operates in a similar fashion to APSS, but it tracks operations as they deviate from plan, to alert commanders about possible ramifications of those deviations. The two systems exercise similar functions in different domains: one anticipates the impact of contingencies and the other anticipates the consequences of operational drift.
The APSS Execution Monitors digest live information to revise plans and expectations on the fly, which is a P function of adjusting as you go along. OpSim Operations Monitors process live information to monitor deviation from plans, which is an A function of scanning for errors. There is a Plan Generator that explores the revised problem spaces for new opportunities and threats, which is an E function of projecting hypothetical possibilities. The I function is headed by a Planning Executive agent which coordinates the activities of the above three agent types as well as models of the outside world (the World View) and communications with the outside world (World Integrator). The Planning Executive is also the level at which human users interact with the system. Thus all elements of the system are integrated into one ensemble by the Planning Executive. The four agent types in this system are described more fully below.
P – Execution Monitors: (APSS) Anticipates the immediate future by attaching to a node in the plan and assessing the difference between actual and planned states for that node. Derives anticipated states by forward simulation along appropriate branches, determining the likelihood of each anticipated state. Execution Monitors essentially explore an option space at a faster rate than the outside world does.
A – Operations Monitors: (OpSim) A dynamic hierarchy of rational agents, each of which monitors a specific aspect of the plan, comparing the actual operation with the planned operation. Significant deviations trigger impact assessment processes, and the top-level OM informs the decision maker when the plan is in jeopardy. Operations Monitors thus generate an error signal or learning signal for a negative feedback process, comparing the actual state of the world to a reference state (the plan).
E – Planner: (APSS) This agent reads the state of a node and uses a Branch Generator to consider possible future actions and produce new relevant branches. The Branch Generator uses simulations, inference mechanisms and genetic algorithms guided by the goal-states or end-states desired by the various parties to the conflict to generate possibilities. A Branch Evaluator then determines how well the terminal node of the new branch accomplishes friendly goals, using simulations and inference. This process generates the option space and the reward or utility (cost/benefit value) of each option.
I – Planning Executive: This agent embodies all four concern specializations, but its role is integrative. It coordinates the flow of tasks and information, allocating certain plan nodes to planners or monitors as required, prioritizing tasks given system resource constraints, and basically cooperating with and controlling all areas of the system as initialized by World View information to make everything work together as an organic and evolving whole.
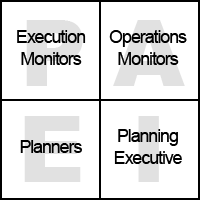
http://paei.wikidot.com/lawless-et-al-organizational-uncertainty-and-planning-tradeo
According to Lawless et al. (Lawless, 2005; Lawless & Grayson, 2004a; Lawless & Grayson 2004b; Lawless et al., 2000a; Lawless et al. 2000b), there are two contrasting models of uncertainty in organizational theory. One derives from game theory and embraces methodological individualism. On this view, organizations are equal to the sum of contributions from the individuals who comprise them. Since the basic unit of analysis is the individual, the way to decrease uncertainty in organizations is to increase communication and coordination between its members.
Lawless et al. also describe a second model of organizational uncertainty, presenting it as a derivative of mathematical physics. On this view, the organization itself can be uncertain, independently of the individuals in it. That is, individuals in an organization might profess complete certainty, but the organization as a whole might be in an extremely uncertain position. Only perturbations of this system expose information about its actual state and the veridicalilty/reliability of its explicit and tacit knowledge.
Cooperation cannot be the solution to this kind of uncertainty. It is actually the problem. When cooperation is easy and routines are undisrupted, minor perturbations can be filtered out of the organization. Internal relationships mutually reinforce each other. The organization can thus drift towards a more uncertain state without realizing it. Information that they are “off course” disappears when cooperation is smooth and easy, buffered from major external disruptions or perturbations. Organizations become insulated by their own prior successes. Lawless writes:
Lawless relates this to the military tactic of "intelligence strikes", forays against the enemy designed specifically to disrupt them in various ways to see how they respond, in order to gain an understanding of the organization of their defence. He also relates it to basic contingency forecasting, where considering problems and conflicts can expose organizational information. His domain of application is defensive military operations, although the same framework is applied to offensive and commercial uncertainty in other papers (Lawless et al., 2000a; Lawless et al., 2000b; Lawless & Grayson, 2004a; Lawless & Grayson, 2004b). In this context, he introduces two paired sources of uncertainty that together make up a fairly significant concern structure model.
Starting from the observations that planning occurs under time pressure and uncertainty, Lawless introduces the Energy/Time uncertainty pairing. Where one is uncertain, we want to be certain about the other. Lawless also posits a Knowledge/Implementation uncertainty pair. We can be uncertain about the adequacy of our plan and the information we are basing it on (Knowledge uncertainty), and we can also be uncertain about our capacity to martial and direct adequate forces in the required manner to actualize those plans (Implementation uncertainty). Again, the less certain we are of one of these factors, the more certain we want to be about the other.
Each of these four types of uncertainty grows in its own distinctive way when systems are left unperturbed. Each one also takes a different kind of perturbation to release it.
P - Execution Uncertainty: When this type of organizational uncertainty grows as a result of effective cooperation, organizational information about effective capacities will only be exposed through conflicts and problems during the execution of plans.
A - Energy Uncertainty: Organizational uncertainty about the accumulation, storage, distribution and burn rate of key or limiting resources can increase under conditions of routine operations. That uncertainty can only be resolved through actual burn rates during the planned operations.
E - Knowledge Uncertainty: Organizational uncertainty can develop regarding the completeness of strategic information and the reliability of intelligence sources. This uncertainty can only be reduced during forays into the field.
I - Time Uncertainty: There may be organizational uncertainty about time dependencies (i.e. who will be where when, and whether that will happen in time to let something else happen, and what everybody else has to do to make that happen, whether all of the parts can come together within critical timeframes or not). This uncertainty can only be reduced by putting the team to the test of an actual performance or exercise.
For the Knowledge/Execution uncertainties, the more time and effort you spend perfecting your knowledge, the smaller your window of opportunity gets for accomplishing urgent goals in the field. The converse of this is "look before you leap". Rushing to implementation too soon with inadequate knowledge will result in extremely costly lessons, exposing the organization's planning and intelligence deficiencies. Around Time/Energy uncertainties, with unlimited energy, the time needed to reach a goal can be lessened dramatically. Conversely, protecting energy stores and restrictively limiting distribution can stretch out the duration of operations, increasing the coordination load and the complexity of distribution activities. There is an effectiveness/efficiency trade-off where effectiveness consumes energy ahead of time and efficiency consumes time ahead of energy.
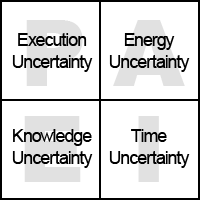
Rowe and Boulgarides (1992) offer a perception-driven theory of decision-making style. Our manner of perceiving and understanding stimuli, on their view, structures our construal of the significance of events. This largely determines how we will respond in decision-making situations.
http://paei.wikidot.com/rowe-boulgarides-decision-style-theory
The dimensions of variance in this decision style theory are cognitive complexity (ambiguity tolerance vs. need for structure) and value orientation (social/human vs. instrumental/task-centered). Crossing these dimensions yields four decision making styles: (1) directive (2) analytical, (3) conceptual, and (4) behavioral, described below in PAEI order.
P - Directive (Low ambiguity tolerance, Task focus): Directive individuals need and value structure. They prefer to make decisions based on clear, undisputed facts and impersonal rules and procedures. They trust their own senses and short, focused reports from others.
A - Analytical (High ambiguity tolerance, Task focus): Analytically minded people can process ambiguity given enough time and information. They rely heavily on abstractions and instrumental logic, and tend to go over all aspects of a problem with a fine-toothed comb, carefully acquiring and organizing large amounts of data. They consider every aspect of a given problem, acquiring information by careful analysis. When presented, their solutions are comprehensive, detailed and very thorough. They may also be innovative if the analysis turned up novel information or supported novel reasoning.
E - Conceptual (High ambiguity tolerance, Social focus): Conceptual decision makers are creative, exploratory, interested in novelty and comfortable taking risks. They are big-picture, creative thinkers who like to consider many different options and possibilities. They gather and evaluate information from many different perspectives, integrating diverse cues and passing intuitive judgments as they work to identify emerging patterns.
I - Behavioral (Low ambiguity tolerance, Social focus): Behavioral decision-makers focus on the feelings and welfare of group members and other social aspects of work. They look to others for information, both explicit information in what others say and implicit information sensed during interactions with them. They evaluate information emotionally and intuitively.
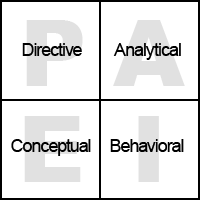
FOUR DECISION MAKING STYLES
http://paei.wikidot.com/scott-bruce-general-decision-making-style-gdms
The General Decision-Making Style test is a psychological instrument developed by Scott and Bruce for two reasons:
1) Their goal was to typify individual differences in decision-making habits and practices, in the domain of career development and vocational behavior studies.
2) The model also emerged inductively out of research plus reviews of the relevant literature, and was subsequently supported by further empirical studies and independent factor analyses. In a sense the model “suggested itself” (Scott & Bruce, 1995).
In their conception, decision-making style is a learned habitual response, resulting in “a habit-based propensity to react a certain way in a specific decision context.” It has been found that people use more than one decision-making style, but one is dominant.
The GDMS underwent a cycle or validation and revision, resulting in the following four decision-making styles:
P – Spontaneous: Sense of immediacy and persistent desire to always finalize decisions as quickly as possible.
A – Rational: comprehensive info search, explicit inventory of alternatives and logical evaluation of options.
E – Intuitive: Alerted by salient details in the flow of information rather than following systematic procedures, more reliance on implicit learning and tacit awareness (“hunches” or “feelings”) as a basis for decisions.
I – Dependent: Resolves uncertainty through consultation, more interested in advice and guidance from others than other styles are.
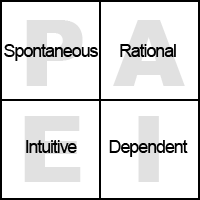